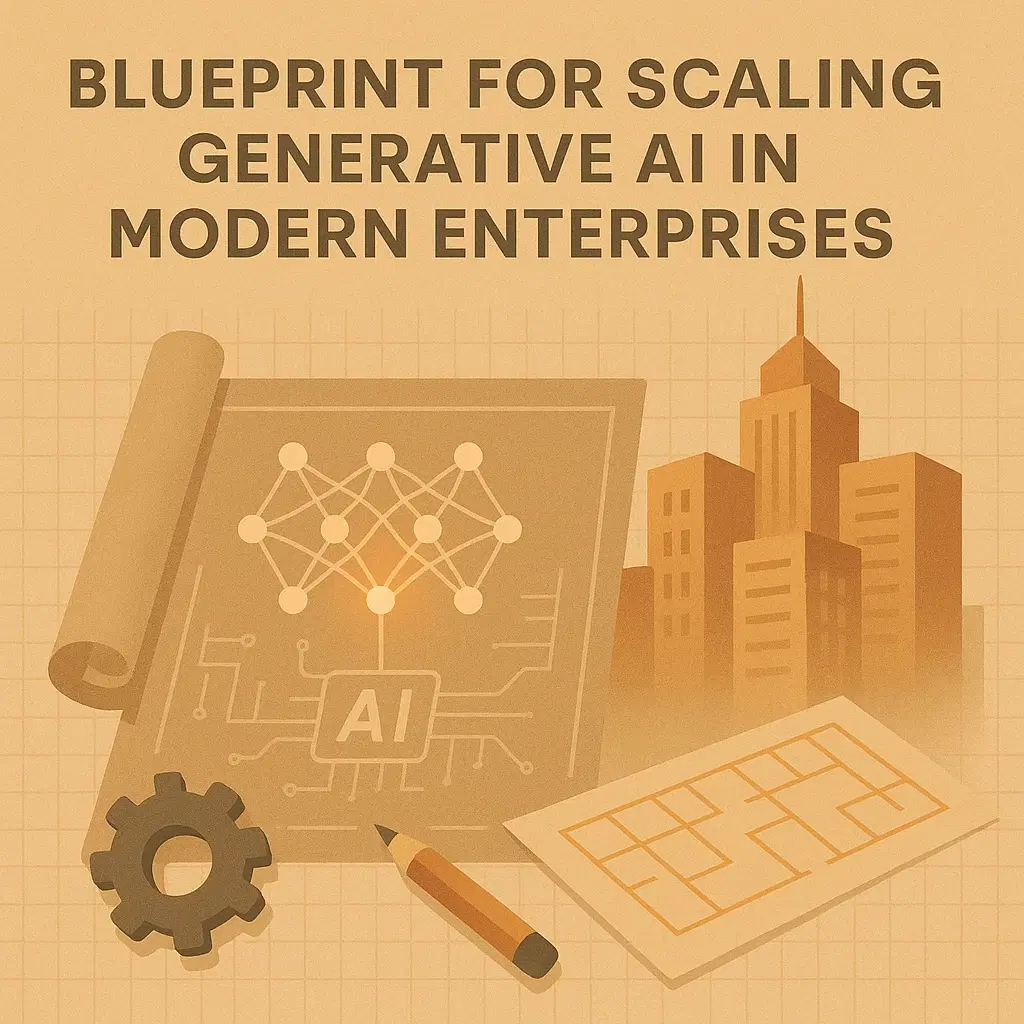
Enterprise AI
•02 min read
The journey to realizing tangible value from Generative AI begins with a well-defined strategy and a thorough assessment of organizational readiness. Jumping into implementation without a clear roadmap can lead to wasted resources, fragmented efforts, and ultimately, failure to achieve strategic objectives. This second installment in our series focuses on the critical steps involved in architecting your enterprise GenAI strategy and evaluating your organization's preparedness for this transformative technology.
Developing a robust GenAI strategy involves several key frameworks and considerations:
Identifying High-Value Use Cases: The starting point is to pinpoint specific business challenges or opportunities where GenAI can deliver significant impact. This requires cross-functional collaboration to understand pain points across different departments and identify areas ripe for automation, augmentation, or innovation. Consider use cases that align with your strategic priorities and offer a clear path to measurable ROI.
Feasibility Assessment: Once potential use cases are identified, a rigorous feasibility assessment is crucial. This involves evaluating the technical viability, potential benefits, associated risks, and resource requirements for each use case. Factors such as data availability, model suitability, and integration complexity should be carefully considered.
Beyond strategic planning, assessing your organization's readiness across several critical dimensions is paramount:
Data Strategy: GenAI, particularly Retrieval-Augmented Generation (RAG) applications, heavily relies on high-quality, accessible data, including unstructured data sources like documents and emails. A thorough assessment of your existing data infrastructure, data governance policies, and data quality is essential. Can you readily access and leverage the data needed for your target use cases?
Talent & Skills: Implementing and managing GenAI solutions requires a skilled workforce. Evaluate your current talent pool and identify gaps in areas such as data science, machine learning engineering, prompt engineering, AI ethics, and governance. Developing a strategy for upskilling existing employees and potentially hiring specialized talent is crucial.
Technology Infrastructure: GenAI workloads can be computationally intensive. Assess your existing compute, storage, and network infrastructure to determine if it can support the demands of GenAI models and applications. Consider the need for specialized hardware like GPUs or TPUs and the scalability of private cloud options.
Governance Framework: Even in the early stages, it's vital to begin thinking about the governance implications of GenAI. This includes initial considerations for data privacy, security, ethical use, and regulatory compliance. Establishing a preliminary framework will help mitigate potential risks as your GenAI initiatives scale.
A crucial element of the readiness phase is adopting a "Start with Evals" approach. Before committing significant resources to scaling a GenAI solution, implement rigorous and structured evaluation processes. This involves defining clear business benchmarks and metrics against which the performance of GenAI models will be measured. Thorough evaluations help ensure that the chosen models are accurate, reliable, and deliver the desired business outcomes before widespread deployment.
Finally, consider the optimal organizational model for driving your GenAI initiatives. Should you establish a centralized Center of Excellence (CoE) to provide expertise and guidance across the organization? Or is a decentralized model, where individual business units are empowered to pursue their own GenAI initiatives, more suitable? The choice often depends on the organization's size, structure, and culture. A hybrid approach, combining centralized expertise with business unit ownership, can also be effective.
Architecting a robust GenAI strategy and thoroughly assessing organizational readiness are foundational steps for successful enterprise adoption. By identifying high-value use cases, evaluating feasibility, addressing critical readiness factors like data, talent, infrastructure, and governance, and prioritizing rigorous evaluations, enterprises can lay a solid groundwork for their GenAI journey. The choice of an appropriate organizational model will further enable effective implementation and scaling. Our next blog will delve into the crucial decisions surrounding implementation roadmaps, platform strategies, and ecosystem integration.